Self-Attention Cloning (SAC)
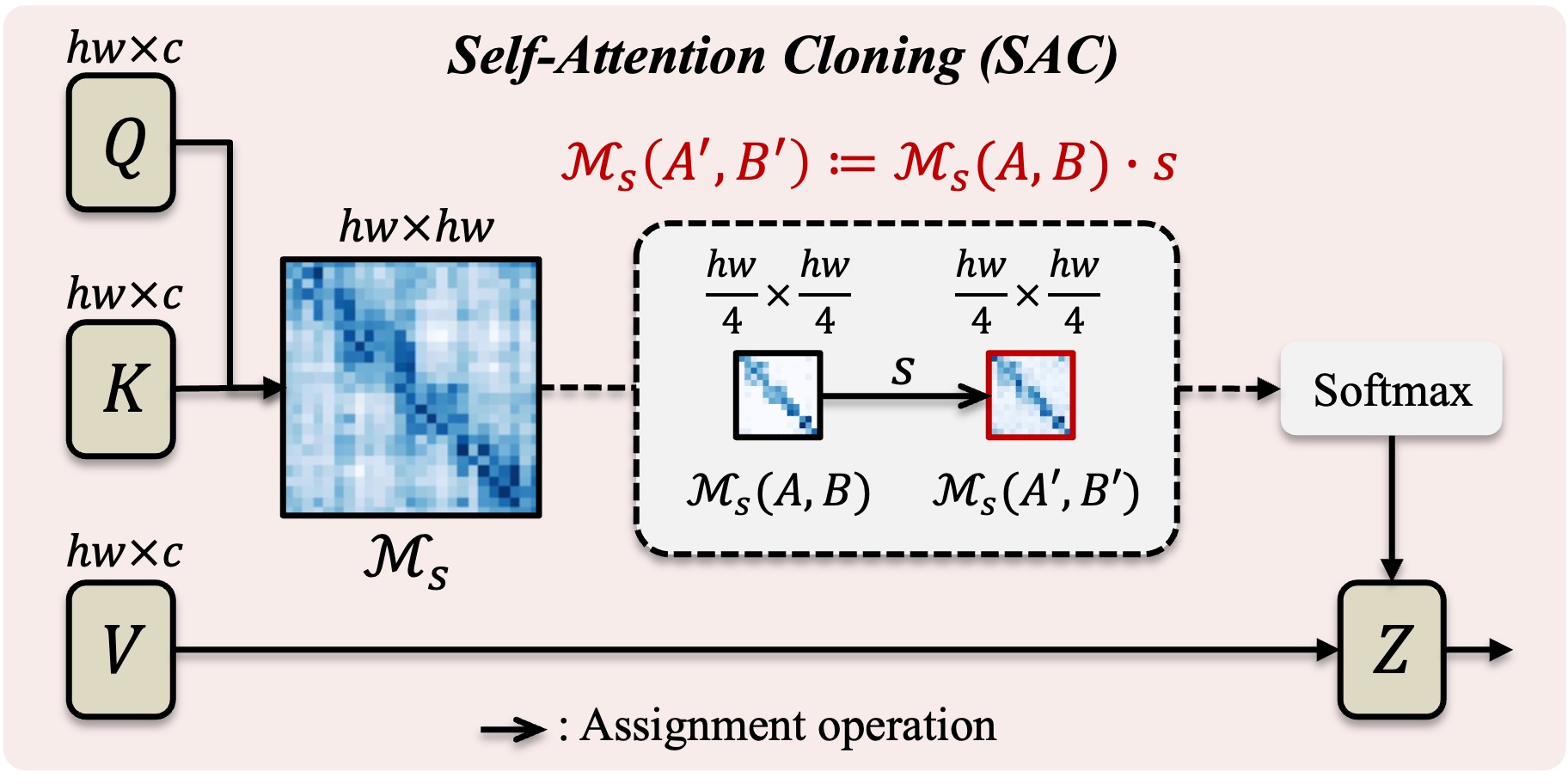
The sub self-attention map \(\mathcal{M}_s(A',B')\) is set as the value of \(\mathcal{M}_s(A,B)\), denoting cloning the relation between \(A\) and \(B\) to that of \(A'\) and \(B'\).
Visual In-Context Learning (ICL) has emerged as a promising research area due to its capability to accomplish various tasks with limited example pairs through analogical reasoning. However, training-based visual ICL has limitations in its ability to generalize to unseen tasks and requires the collection of a diverse task dataset. On the other hand, existing methods in the inference-based visual ICL category solely rely on textual prompts, which fail to capture fine-grained contextual information from given examples and can be time-consuming when converting from images to text prompts.
To address these challenges, we propose Analogist, a novel inference-based visual ICL approach that exploits both visual and textual prompting techniques using a text-to-image diffusion model pretrained for image inpainting. For visual prompting, we propose a self-attention cloning (SAC) method to guide the fine-grained structural-level analogy between image examples. For textual prompting, we leverage GPT-4V's visual reasoning capability to efficiently generate text prompts and introduce a cross-attention masking (CAM) operation to enhance the accuracy of semantic-level analogy guided by text prompts.
Our method is out-of-the-box and does not require fine-tuning or optimization. It is also generic and flexible, enabling a wide range of visual tasks to be performed in an in-context manner. Extensive experiments demonstrate the superiority of our method over existing approaches, both qualitatively and quantitatively.
Overview of the proposed Analogist. A visual demonstration is defined by an example pair \(A\) (woman holding a cat) and \(A'\) (the same woman holding a tiger). Given a new image \(B\) (another cat), we format these three images into a \(2 \times 2\) grid and tackle this problem by fill the missing image via a pretrained Stable Diffusion inpainting model. The images are arranged into a \(2 \times 2\) grid and feed into a pretrained inpainting model. We employ GPT-4V to provide a proper text description (i.e., "close-up of a tiger's face") to further guide the inpainting process. During the process of model inference, Self-Attention Cloning (SAC) and Cross-Attention Masking (CAM) are introduced to encourage the model concentrate on the visual and textual prompts
The sub self-attention map \(\mathcal{M}_s(A',B')\) is set as the value of \(\mathcal{M}_s(A,B)\), denoting cloning the relation between \(A\) and \(B\) to that of \(A'\) and \(B'\).
The sub cross-attention map between text embedding and regions \(A\), \(A'\), and \(B\) are set to zero, making the semantic guidance more focused on region \(B'\).
@article{gu2024analogist,
title = {Analogist: Out-of-the-box Visual In-Context Learning with Image Diffusion Model},
author = {GU, Zheng and Yang, Shiyuan and Liao, Jing and Huo, Jing and Gao, Yang},
journal = {ACM Transactions on Graphics (TOG)},
year = {2024},
}